Spring '22 Department Seminars with Dr. Hatice Gökcan
Title: Prediction of Protein pa with Representation Learning
Speaker: Dr. Hatice Gökcan
Department of Chemistry, Carnegie Mellon University
Pittsburgh, United States
Date: May 10th, 2022, Tuesday
Time: 17:30
Place: Zoom
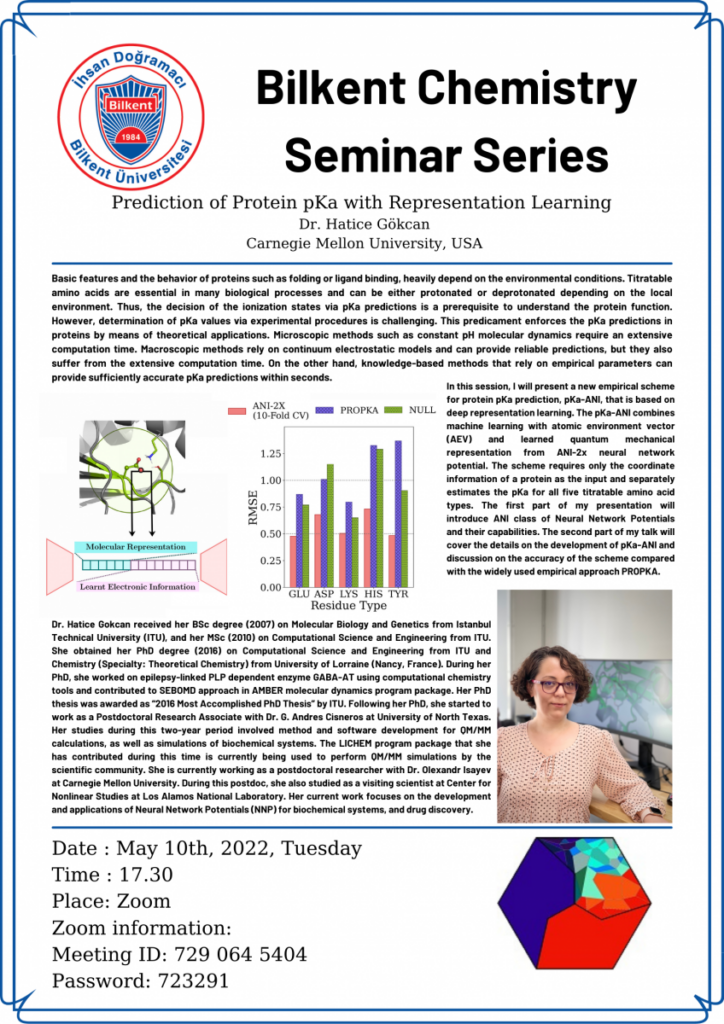
Abstract:
Basic features and the behavior of proteins such as folding or ligand binding, heavily depend on the environmental conditions. Titratable amino acids are essential in many biological processes and can be either protonated or deprotonated depending on the local environment. Thus, the decision of the ionization states via pka predictions is a prerequisite to understand the protein function. However, determination of pKa values via experimental procedures is challenging. This predicament enforces the pKa predictions in proteins by means of theoretical applications. Microscopic methods such as constant pH molecular dynamics require an extensive computation time. Macroscopic methods rely on continuum electrostatic models and can provide reliable predictions, but they also suffer from the extensive computation time. On the other hand, knowledge-based methods that rely on empirical parameters can provide sufficiently accurate pKa predictions within seconds.
In this session, I will present a new empirical scheme for protein pka prediction, pKa-ANI, that is based on deep representation learning. The pKa-ANI combines machine learning with atomic environment vector (AEV) and learned mechanical (AEY)quantum representation from ANI-2x neural network potential. The scheme requires only the coordinate information of a protein as the input and separately estimates the pKa for all five titratable amino acid types. The first part of my presentation will introduce ANI class of Neural Network Potentials and their capabilities. The second part of my talk will cover the details on the development of pKa-ANI and discussion on the accuracy of the scheme compared with the widely used empirical approach PROPKA.
Short Biography of the Speaker:
Dr. Hatice Gokcan received her BSc degree (2007) on Molecular Biology and Genetics from Istanbul Technical University (ITU), and her MSc (2010) on Computational Science and Engineering from ITU. She obtained her PhD degree (2016) on Computational Science and Engineering from ITU and Chemistry (Specialty: Theoretical Chemistry) from University of Lorraine (Nancy, France). During her PhD, she worked on epilepsy-linked PLP dependent enzyme GABA-AT using computational chemistry tools and contributed to SEBOMD approach in AMBER molecular dynamics program package. Her PhD thesis was awarded as “2016 Most Accomplished PhD Thesis” by ITU. Following her PhD, she started to work as a Postdoctoral Research Associate with Dr. G. Andres Cisneros at University of North Texas. Her studies during this two-year period involved method and software development for QM/MM calculations, as well as simulations of biochemical systems. The LICHEM program package that she has contributed during this time is currently being used to perform QM/MM simulations by the scientific community. She is currently working as a postdoctoral researcher with Dr. Olexandr Isayev at Carnegie Mellon University. During this postdoc, she also studied as a visiting scientist at Center for Nonlinear Studies at Los Alamos National Laboratory. Her current work focuses on the development and applications of Neural Network Potentials (NNP) for biochemical systems, and drug discovery.